News Story
Artificial Intelligence solutions for commercial adoption of solar cells
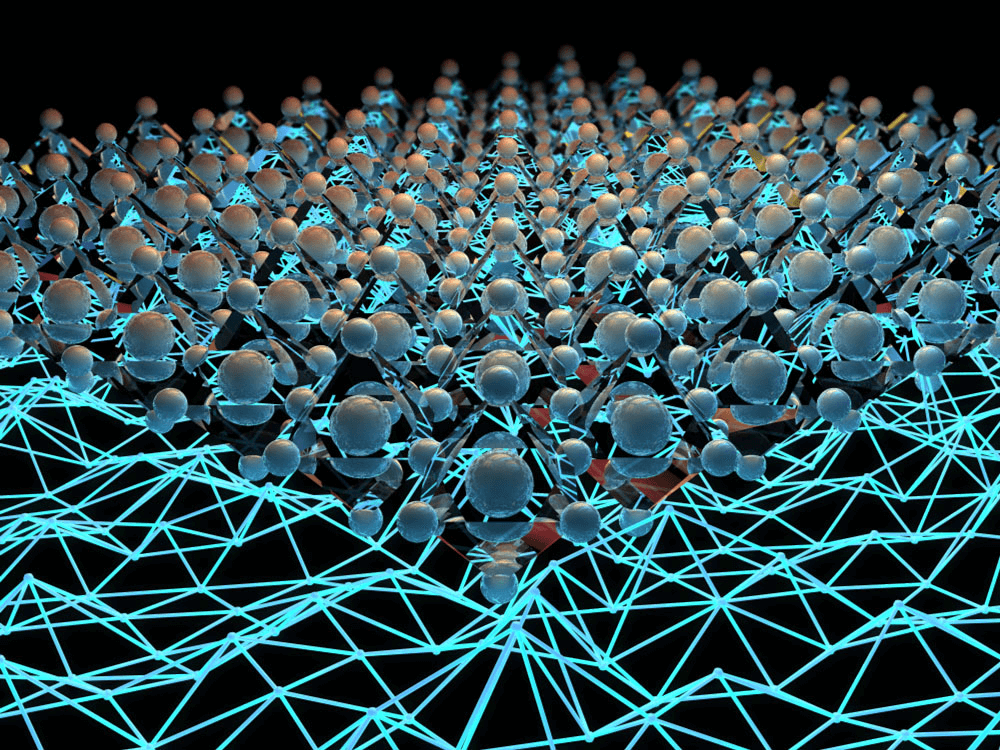
Marina Leite, University of Maryland Assistant Professor in Materials Science and Engineering, and her team of researchers grace the cover of the February 20th issue of Joule magazine for their pioneering work on artificial intelligence solutions using solar cell materials.
High-performing and low-cost photovoltaics (PV) are critical to the continued adoption of renewable energy sources. While promising, perovskite solar materials show a dynamic optoelectronic response when exposed to water, oxygen, bias, temperature, or light that severely impacts their performance, preventing commercialization of these solar cells. According to Leite, by reframing perovskites' optoelectronic transiency within the context of recovery rather than degradation, they are able to highlight research opportunities and the artificial intelligence solutions needed for the commercial adoption of these promising solar cell materials.
Hybrid organic-inorganic perovskite (HOIP) photovoltaic (PV) devices are an emerging technology with record power conversion efficiency. But the effects of water and oxygen as well as temperature and light can often hinder the efficiency. The research team believes that identifying, understanding, and controlling the influence of each one of these factors (as well as their combined effects) toward the stability of HOIPs from the macro- to the nanoscale will continue to be a major thrust in the research community.
Macro- to nanoscale characterization can complement one another to correlate structural, electrical, optical, and chemical properties throughout the perovskite 3R cycle. More importantly, these tools will allow the scientific community to identify the most influential environmental parameters, as well as the cutoff between recovery and degradation. Using this information, advanced computational frameworks based on ML could be quickly developed that maximize overall long-term power output, minimizing material degradation. AI strategies facilitating rapid knowledge transfer could ultimately enable reliable perovskite solar cells.
First author and UMD MSE student, John Howard, did some preliminary work through his Harry K. Wells Fellowship awarded by the Maryland Energy Innovation Institute (MEI2). His research focused on identifying degradation mechanisms in perovskite solar cells. Mr. Harry K. Wells established an endowment to support engineering graduate students at the University of Maryland (UMD) pursuing new research in the field of sustainable energy generation and/or storage.
Full publication: Machine Learning for Perovskites' Reap-Rest-Recovery CycleJohn M. Howard,Elizabeth M. Tennyson,Bernardo R.A. Neves,and Marina S. Leite,Volume 3, Issue 2, 20 February 2019, Pages 325-337, https://doi.org/10.1016/j.joule.2018.11.010
Published February 27, 2019